How to Mine Financial Data for Hidden Insights
Author Prexisio
Published on April 14, 2025
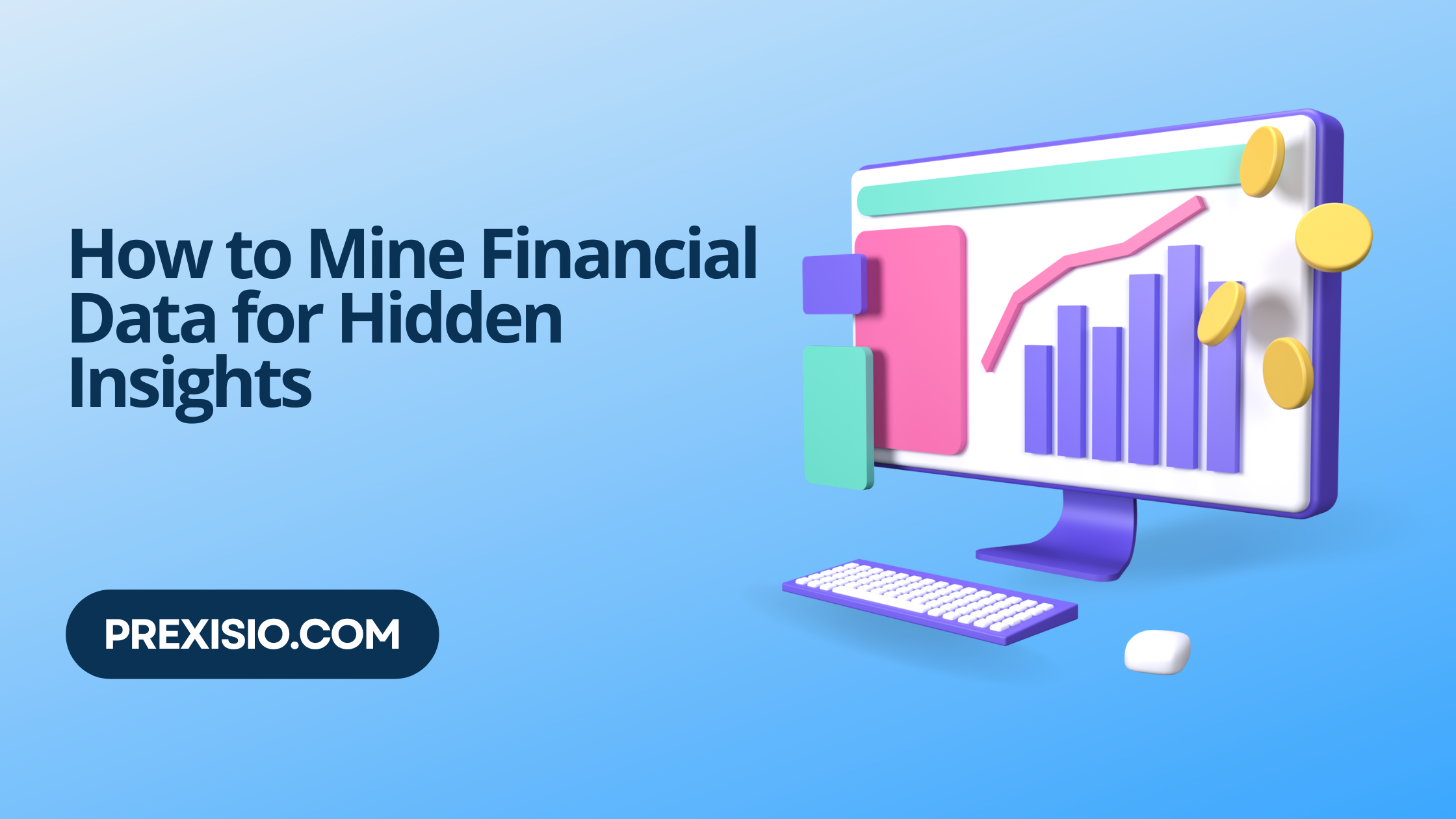
Introduction
Small-to-medium businesses (SMBs) are sitting on a goldmine of financial data. However, without the right tools and techniques, much of this data remains underutilized—its valuable insights hidden in plain sight. Modern business leaders are discovering that mining financial data using AI and SQL-based methods can unlock these hidden insights to drive smarter decisions, greater efficiency, and higher profits. Research shows that CFOs who embrace data-driven decision-making achieve 19% higher profitability than their peers. This comprehensive guide explores how AI and SQL can turn raw financial data into actionable intelligence, empowering business managers, CFOs, and business decision-makers to lead with confidence.
Why It Matters
Data-driven decision-making in finance is no longer a luxury—it’s a necessity. U.S. businesses are increasingly leveraging analytics and AI to guide financial strategy. According to a 2024 survey, 91% of SMBs using artificial intelligence report that it boosts their revenue. Similarly, PwC’s research finds that over a quarter of finance departments already use AI for forecasting, with many also applying AI to accounts payable, receivables, and predictive analytics. The momentum is clear: 75% of SMBs are at least experimenting with AI, and among high-growth SMBs, that number jumps to 83%. Those who invest in data and AI capabilities are reaping tangible rewards, from faster growth to improved profitability. Conversely, organizations that delay adopting data-driven practices risk falling behind the competition. The message for CFOs and business decision makers is evident—harnessing financial data isn’t just about crunching numbers; it’s about staying competitive in this AI era.
Key Benefits
How exactly can data mining translate into business value for a mid-market or SMB organization? Here are several practical use cases where AI and SQL-based financial data mining make a meaningful impact:
Intelligent Cash Flow Forecasting: Cash flow is the lifeblood of any business, yet forecasting cash positions is often a challenge. AI-powered models can accurately forecast future cash flow by mining historical cash inflows and outflows (from AR/AP aging reports, sales trends, and expense schedules). For example, an AI model might analyze seasonal sales patterns, customer payment behaviors, and upcoming payables to predict when you might face a cash shortfall. This allows CFOs to take proactive action—such as adjusting payment terms or securing a line of credit in advance. Companies using such AI-driven forecasting have seen significant improvements in accuracy (in one case, forecast variance was reduced by 40% when AI tools were applied to budgeting). The insight: better forecasts mean fewer surprises, reduced risk of cash crunches, and confidence to make growth investments at the right time.
Expense Optimization and Cost Control: Financial data mining can reveal hidden patterns in your expenses that lead to cost savings. SMBs often have sprawling expense categories—from office supplies and subscriptions to supplier contracts. Using SQL queries, a business can consolidate all expense data and then apply analytics to spot anomalies or trends. For instance, you might discover a spending pattern where a particular vendor’s costs have crept up 10% every quarter, or find duplicate software subscriptions being paid across different departments. AI algorithms can flag outliers (why did travel expenses spike this month?) and even categorize spend more accurately through pattern recognition. Companies directly improve their bottom line by acting on these insights—renegotiating contracts, eliminating wasteful spending, or streamlining suppliers. Data mining for expense insights is essentially a form of profit mining: every dollar saved goes straight to profit. It’s not trivial either; a Deloitte survey noted that 35% of finance teams already use AI for process automation (including spend management), reflecting how important cost optimization has become in the age of analytics.
Customer Profitability and Segmentation: Not all revenue is equal. Some customers or product lines might be far more profitable than others once you account for discounts, service costs, or returns. By integrating sales data (revenue per customer/product) with cost data (direct costs, support cases, returns, etc.), mid-market firms can determine which customers, markets, or products deliver the highest margin versus which might even be unprofitable. SQL can help aggregate customer-level P&Ls, and AI can cluster customers into segments based on profitability drivers. For example, you might learn that small businesses in a certain region have high sales but also high support costs, resulting in lower net margins—a signal to adjust pricing or service models. Another insight could be identifying your “80/20 rule” — the 20% of customers or products driving 80% of profit — so you can focus retention and upselling efforts there. One McKinsey analysis found data-driven resource allocation like this can boost revenue significantly. In practice, an SMB could use these insights to refine its strategy (perhaps focusing marketing on a high-margin industry segment, or discontinuing a product that consistently loses money). The result is a more efficient allocation of resources and higher overall profitability driven by facts, not hunches.
Working Capital and Risk Management: Financial data mining also plays a crucial role in managing risk and improving operational efficiency. For instance, analyzing accounts receivable data may reveal hidden patterns in late payments—perhaps certain industries or client types are slower to pay. An AI model could use those patterns to predict which invoices are likely to go past due, allowing the finance team to intervene early (e.g., send reminders or adjust credit terms). In one survey, companies that implemented advanced working capital analytics achieved 15% better cash conversion cycles than those that didn’t. Similarly, mining payables and inventory data can highlight opportunities to optimize working capital: maybe you discover that if you pay a key supplier a week early, you consistently can take a 2% early-pay discount, boosting margins. On the risk side, anomaly detection algorithms can run through general ledger entries to flag irregular transactions for review (critical for fraud prevention and compliance monitoring). For example, an unexpected spike in expenses in a rarely-used account, or transactions just below approval thresholds, could be caught by AI that learns what “normal” looks like in your books. By surfacing these red flags, CFOs can tighten internal controls and reduce the risk of financial errors or fraud. The payoff is twofold: efficiency gains (like one company that cut its days sales outstanding by 21% through automated collections prompts) and risk reduction by catching issues before they escalate.
These use cases illustrate a common theme: by digging into data, we uncover actionable insights that were previously hidden. Each insight, in turn, suggests a concrete action—whether it’s adjusting a forecast, cutting a cost, reallocating resources, or mitigating a risk. Importantly, these examples are highly relevant to mid-sized firms and SMBs; you don’t need a Fortune 500 budget to apply these techniques. Even a small finance team with the right approach can leverage AI-driven analysis on their SQL-accessible data to find efficiencies and growth opportunities. The key is to start with a clear question or problem (e.g., “How can we improve cash flow?” or “Which customers should we focus on?”) and then let the data guide your decision-making.
How-To Guide
There are several techniques for mining financial data for hidden insights, but for me, two technologies stand out in this AI era: Artificial Intelligence (AI) and SQL (Structured Query Language). Each plays a distinct but complementary role:
SQL – The Data Excavator: SQL is the workhorse for extracting, querying, and aggregating data from financial databases. Virtually all ERP and accounting systems run on SQL databases, making SQL queries essential for retrieving transaction records, joining tables (e.g. linking sales data with expense data), and performing initial analysis. With SQL, a finance team can pull a report of, say, monthly revenue by product across regions, or identify all invoices above a certain value. In financial data mining, SQL helps dig into large datasets efficiently, ensuring you work with the correct information before deeper analysis. For example, a CFO might use SQL to query the ERP for all outstanding receivables and their ages, laying the groundwork for an AI model that predicts which invoices are at risk of late payment.
AI – The Insight Generator: Artificial intelligence encompasses machine learning algorithms and advanced analytics techniques that learn patterns from historical data and make predictions or categorizations. AI can uncover trends and anomalies beyond what manual analysis or standard reports reveal. Predictive models can forecast cash flow or revenue with greater accuracy, while anomaly detection algorithms can scan thousands of transactions to flag unusual entries that might indicate fraud or errors. AI can even leverage natural language processing to analyze text-based financial data (like contracts or invoices) for risk factors. By applying AI to the data that SQL pulls together, companies move from basic historical reporting to forward-looking insights—such as predicting next quarter’s sales, identifying cost drivers, or assessing the financial impact of different scenarios.
Together, SQL and AI form a powerful one-two punch. Think of SQL as preparing and structuring the data (the “mining” of raw ore) and AI as the analytical engine that extracts valuable insights from that ore. Modern financial analytics tools often integrate both: for instance, Prexisio's chat with your data platform uses SQL under the hood to clean and transform your data, then apply AI-driven analytics (like automatic trend detection or forecast suggestions) on top. The result is a streamlined process where you can ask complex questions (“Which factors most impact our cash flow volatility?”) and get data-backed answers that would have been impossible to find manually.
Common Financial Data Sources to Use
Mining financial data effectively starts with knowing where to dig. SMBs typically have multiple data sources that collectively hold the keys to hidden insights. Key sources include:
ERP and Accounting Systems: These are the central repositories of financial transactions—your general ledger, accounts payable/receivable, invoices, purchase orders, inventory costs, and more. ERP systems like Oracle NetSuite, Microsoft Dynamics, or SAP Business One (and accounting software like QuickBooks or Sage Intacct for smaller firms) capture day-to-day financial events. They are a treasure trove for data mining: every sale, expense, and journal entry lives here. For example, an ERP’s data can help identify revenue trends, expense patterns, or budget variances over time.
Financial CRMs and Sales Systems: Many SMBs use customer relationship management (CRM) systems or sales platforms that track customer orders, pipelines, and billing. These systems (like Salesforce, HubSpot, or industry-specific CRMs) provide the revenue side of the picture. By mining CRM data alongside accounting data, companies can derive customer-level profitability (e.g. linking customer acquisition cost and lifetime value) or forecast revenue based on pipeline health. For instance, if your CRM shows a surge in leads and your accounting data shows seasonally strong conversion rates, an AI model could project an upcoming revenue boost—allowing you to plan inventory or staffing accordingly.
Operational Systems (Inventory, Payroll, etc.): Financial insights don’t only reside in pure “finance” software. Payroll and HR systems contain data on labor costs, overtime, and productivity that impact financial performance. Inventory and supply chain systems hold data on cost of goods, turnover rates, and supplier payments that affect cash flow. Mining these data sources can reveal insights like which products tie up the most cash in inventory or how staffing costs correlate with project profitability. For example, analyzing payroll data might show overtime spikes in certain months, prompting a staffing adjustment to control costs.
Spreadsheets and External Data: Let’s not forget the ubiquitous Excel or Google Sheets that many SMB finance teams still rely on. These often contain manually compiled reports, forecasts, or supplemental data (like departmental budgets or scenario analyses). While spreadsheets can be error-prone and siloed, they might hold historical knowledge that can be integrated into larger analyses. Additionally, external data sources such as market benchmarks, economic indicators, or industry reports can be merged with internal financial data to add context. For instance, comparing your sales trends with economic data (like consumer spending indices) could help explain variances or refine your forecasts.
Each of these sources on its own is valuable, but the real magic happens when you combine them. A holistic data mining effort might pull accounts receivable info from the ERP, customer purchase history from the CRM, and collection notes from a spreadsheet or support system to train an AI model that predicts payment defaults. By breaking down data silos and merging datasets, hidden correlations emerge. (Is a certain customer’s late payment actually foreshadowed by a drop in their monthly orders? Do longer delivery times correlate with higher return rates and lost revenue?) Many mid-sized companies find that once they unify their data, they gain a 360° view of their business that was never possible before—confirming the adage that integrated data leads to integrated insights
Pro Tips
Uncovering hidden insights is only half the way—the ultimate goal is to translate insight into action. For business managers and CFOs, this means establishing a process where analytical findings inform decision-making in a tangible way. Here’s how to ensure your data mining efforts lead to real-world improvements in decision-making, efficiency, profitability, and risk reduction:
1. Validate and Contextualize the Insights: Not every pattern your AI model finds will make sense at first glance. Before rushing to act, sanity-check the insight. Does a flagged trend or correlation have a logical business explanation? Engage domain experts to interpret why, for example, a certain expense is spiking or why a forecast is higher than usual. Validation is critical to avoid false alarms or overreacting to anomalies that turn out to be one-time events. By grounding data insights in business context, you ensure you’re acting on meaningful information. This is where human experience pairs with data science—finance teams should discuss insights in management meetings, bringing in context that algorithms might not know (like a recent marketing campaign that explains a sales jump).
2. Quantify the Impact: Tie the insight to key performance indicators (KPIs) or financial outcomes. If data mining reveals an opportunity to save costs, estimate how much savings are possible. If it identifies a risk, quantify the potential impact if left unaddressed. For instance, if your analysis finds an uptick in late payments, calculate how that affects cash flow or bad debt expense. Quantifying the value of an insight helps prioritize actions. Knowing the potential impact (in dollars, days, or percentage improvement) creates urgency and buy-in to act on the insight.
3. Make Data-Driven Decisions (and Policies): Use the insights as a foundation for concrete decisions or policy changes. If analysis shows a particular product line is underperforming, the decision might be to discontinue it or redesign it. If an AI model predicts a cash shortfall next quarter, the decision could be to secure additional financing or delay a planned expense. The key is to integrate these data-driven insights into your strategic planning and daily management. Some decisions will be one-off (e.g., a cost-cutting measure), while others might become new policies or processes. For example, seeing consistent success in a predictive model for collections might lead a CFO to implement a new credit risk policy or an automated dunning process for late invoices. By codifying learnings into company policy, you create a loop where data mining continuously informs how the business is run.
4. Monitor Outcomes and Refine: Once an action is taken, close the loop by monitoring the results. Did the anticipated cost savings actually materialize after cutting that expense? Is the improved cash flow forecast holding true after implementing new collection procedures? Tracking outcomes measures success and provides new data to feed back into your models, creating a continuous improvement cycle. This practice helps in refining models and assumptions. Maybe your first forecast model was 80% accurate; after some tweaks and more data, you push it to 90% accuracy. Many businesses find that this iterative approach, supported by timely data, fosters a culture of continuous improvement. Data-driven organizations often develop a competitive advantage here, as they learn and adapt faster than those relying on gut feel. Leaders should encourage teams to view insights as catalysts for experiments—test a change, measure it, and scale up what works. Over time, this tight feedback loop between data insight and action can improve efficiency (for instance, it is possible that automated processes can shave 4 days off the month-end close, freeing up time for value-added analysis) and enhance profitability.
In summary, interpreting and acting on financial data insights is about making your organization smarter and faster. Smarter, because decisions are based on evidence and likely outcomes. Faster, because real-time dashboards and AI alerts can prompt immediate responses to emerging issues or opportunities. When every major decision—be it a budget change, an investment, or a risk mitigation plan—is supported by robust data analysis, business leaders can move forward with confidence. This is the essence of data-driven financial management: better decisions, made sooner, with clearer visibility into their consequences.
Data Quality, Governance, and Automation: Laying the Foundation
No discussion of financial data mining is complete without addressing the foundation that makes it all possible: data quality, governance, and automation. These are the unsung heroes enabling effective data mining. If AI and SQL are the powerful excavators and analyzers, then data quality and governance are the solid ground and map that ensure you dig in the right place, and automation is the machinery that keeps the operation running smoothly. Here’s why they matter:
Data Quality – Trustworthy Data or Nothing: The value of any insight is only as good as the data behind it. “Garbage in, garbage out” holds especially true in finance. Inaccurate or incomplete data can lead to flawed analysis and misguided decisions. Unfortunately, poor data quality is a widespread challenge—Gartner estimates that bad data costs organizations an average of $12.9 million annually. While that figure often applies to larger enterprises, the spirit holds for SMBs: bad data leads to costly mistakes (in missed opportunities or direct losses) regardless of company size. Common data quality issues include duplicate records, inconsistent entry formats, missing values, or data that’s simply outdated. For example, if two departments use slightly different coding for expenses, an analysis of total spend might double-count or omit certain figures. To combat this, companies need to invest in data cleaning and validation. This might mean periodic audits of financial data, using tools that de-duplicate and standardize records, and setting up controls in systems to enforce valid entries. High data quality ensures that when you mine your financial data, you’re striking gold, not fool’s gold.
Data Governance – Single Source of Truth: Data governance refers to the processes and policies that manage data availability, usability, consistency, and security. In a financial context, this means establishing clear ownership of data (who manages the chart of accounts? who is responsible for sales data?), setting definitions (what exactly qualifies as “Customer Acquisition Cost”?), and ensuring compliance with regulations (like GAAP, SOX, or data privacy laws). Good data governance prevents the scenario of different versions of the truth. Many mid-market firms struggle with siloed information where, say, the finance team reports one profit figure and the sales team reports another for the same period due to differing data sources or assumptions. Governance practices, such as maintaining a central data dictionary and coordinating cross-department data reconciliation, eliminate these discrepancies. Modern tools can help by providing data lineage (tracking where each data point comes from) and access controls (so the right people have the right data). Governance also entails integrating systems so that everyone is drawing from a unified database or data warehouse.
Automation – From Data Collection to Reporting: Automation ties everything together by handling the repetitive, time-consuming aspects of data management and analysis. Many finance teams still spend inordinate amounts of time each month manually exporting data, merging spreadsheets, fixing formula errors, or preparing slide decks for reporting. Not only is this inefficient, it’s prone to human error. Studies show 73% of companies waste time on manual tasks that AI could automate. Automation, whether through specialized ETL (Extract, Transform, Load) data pipelines, robotic process automation (RPA) scripts, or built-in features of BI tools, can dramatically reduce this burden. For example, rather than an analyst manually reconciling financial data from two systems, an automated workflow can pull data from both, merge it, and even highlight mismatches for review. Or instead of hand-crafting a report each week, a dashboard could be set up once and then auto-refresh with live data. Automating data ingestion and reporting frees up your finance talent to focus on analysis and interpretation rather than data wrangling. Automation also improves timeliness—imagine having daily cash flow updates instead of waiting for month-end reports. Many mid-market firms are adopting automation as a force multiplier; CFO surveys show 67% are prioritizing investments in financial automation and predictive analytics tools.
Paying attention to data quality, governance, and automation establishes a strong foundation for any financial data mining initiative. Think of it like preparing a mine before extraction: you clear the debris (clean your data), map the tunnel (govern your data), and bring in modern machinery (automate processes) to ensure a smooth operation. Doing so enables your AI and SQL tools to work at their full potential. The result is a virtuous cycle: high-quality, well-governed data feeds better analysis; better analysis drives confident action; and automated feedback loops continuously improve both the data and the decisions made from it. For SMBs and mid-sized businesses, these practices level the playing field, allowing you to punch above your weight in analytics without needing the massive data teams that big corporations have. It’s about working smarter, not harder—letting the systems handle the grunt work while your team focuses on strategy and insight.
Conclusion
Financial data is one of a business's most powerful assets—if you know how to mine it for insights. By leveraging AI and SQL-based data mining techniques, even resource-constrained mid-market companies can uncover hidden patterns that drive better decision-making, greater efficiency, higher profitability, and reduced risk. The trends of 2024–2025 make it clear that data-driven financial management is becoming standard practice, from AI-enhanced forecasting in the finance department to real-time dashboards that guide day-to-day decisions. Forward-looking business managers and CFOs are investing in the tools, skills, and governance needed to turn their financial databases into decision-making engines.
The payoff for those who succeed is significant: more accurate forecasts, optimized costs, improved cash flow, and strategies grounded in facts rather than guesswork. As we’ve seen, companies embracing these practices have already realized performance boosts like double-digit efficiency gains and profit increases. The roadmap is here—identify your key data sources, apply AI and analytics to generate insights, and build the internal processes to act on those insights quickly and effectively.
Ready to unlock the hidden insights in your financial data? Prexisio stands at the forefront of this data-driven finance revolution. As a thought leader in AI-powered business intelligence, Prexisio helps SMBs and mid-market firms build the right data infrastructure, apply advanced analytics, and automate workflows to transform raw data into real results. Don’t let valuable insights stay buried in your databases. Struggling with siloed data or unsure how to start with AI in finance? Prexisio can help you harness your financial data for smarter decision-making. Contact us or schedule a consultation and let our experts guide you in turning your financial data into a strategic advantage. Your data already holds the answers—Prexisio will help you find them and put them into action.